S-ATLAS Probabilistically Correct Language-based Multi-Robot Planning using Conformal Prediction
IEEE Roboics and Automation Letters (RA-L)
Abstract
This paper addresses task planning problems for language-instructed robot teams. Tasks are expressed in natural language (NL), requiring the robots to apply their capabilities at various locations and semantic objects. Several recent works have addressed similar planning problems by leveraging pre-trained Large Language Models (LLMs) to design effective multi-robot plans. However, these approaches lack performance guarantees. To address this challenge, we introduce a new distributed LLM-based planner, called S-ATLAS for Safe plAnning for Teams of Language-instructed AgentS, that is capable of achieving user-defined mission success rates. This is accomplished by leveraging conformal prediction (CP), a distribution-free uncertainty quantification tool in black-box models. CP allows the proposed multi-robot planner to reason about its inherent uncertainty in a distributed fashion, enabling robots to make individual decisions when they are sufficiently certain and seek help otherwise. We show, both theoretically and empirically, that the proposed planner can achieve user-specified task success rates, assuming successful plan execution, while minimizing the overall number of help requests. We provide comparative experiments against related works showing that our method is significantly more computational efficient and achieves lower help rates. The advantage of our algorithm over baselines becomes more pronounced with increasing robot team size.
Demonstration Videos (under AI2THOR environment)
Framework Structure
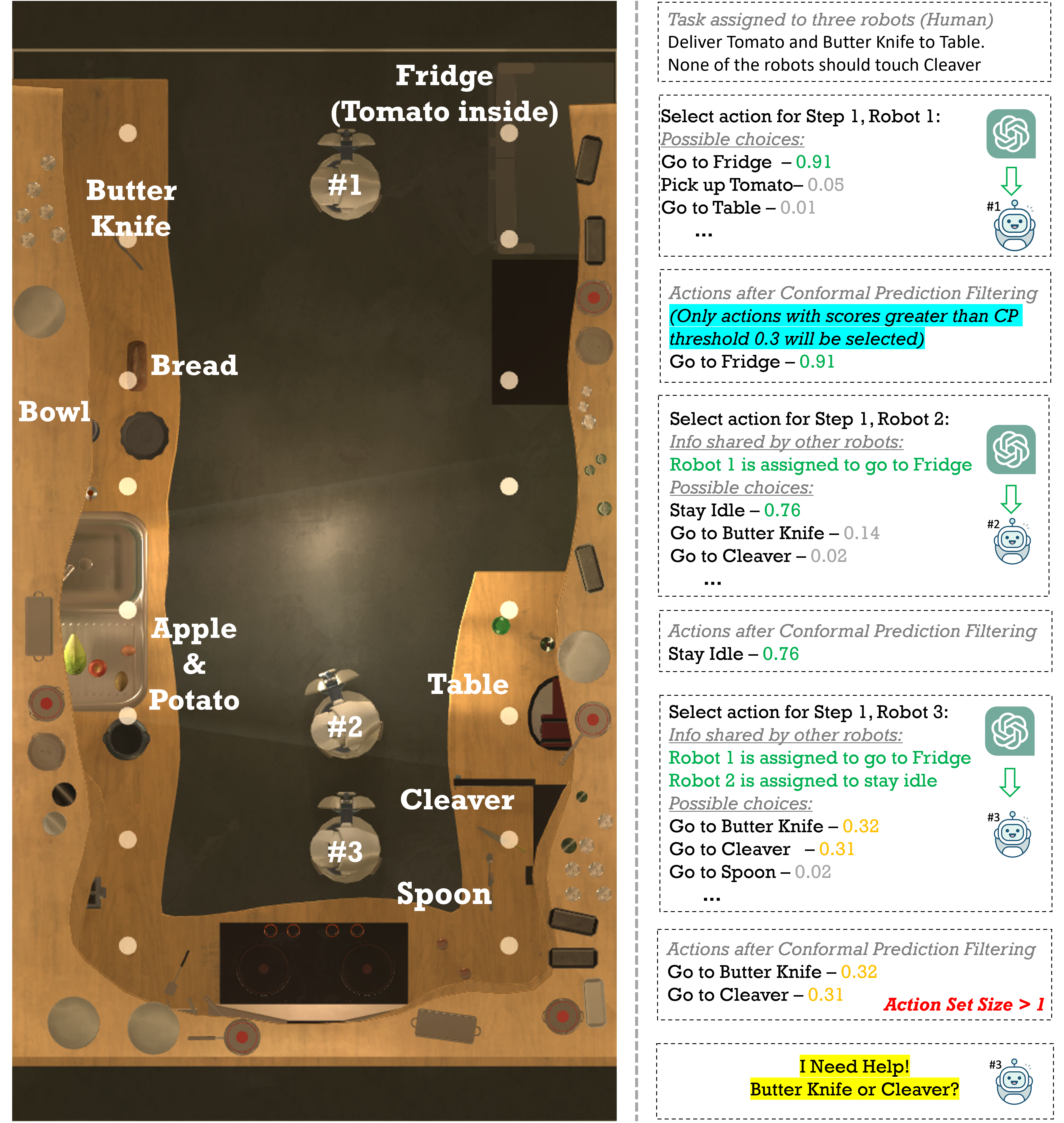
We propose a distrbuted planner for language-instructed multi-robot systems being capable of achieving user-specified mission success rates. The robots are delegated to pre-trained Large Language Models (LLMs), enabling them to select actions while coordinating in a conversational manner. Our LLM-based planner reasons about its inherent uncertainty, using conformal prediction, in a decentralized fashion. This capability allows the planner to determine when and which robot is uncertain about correctness of its next action. In cases of high uncertainty, the respective robots seek assistance.
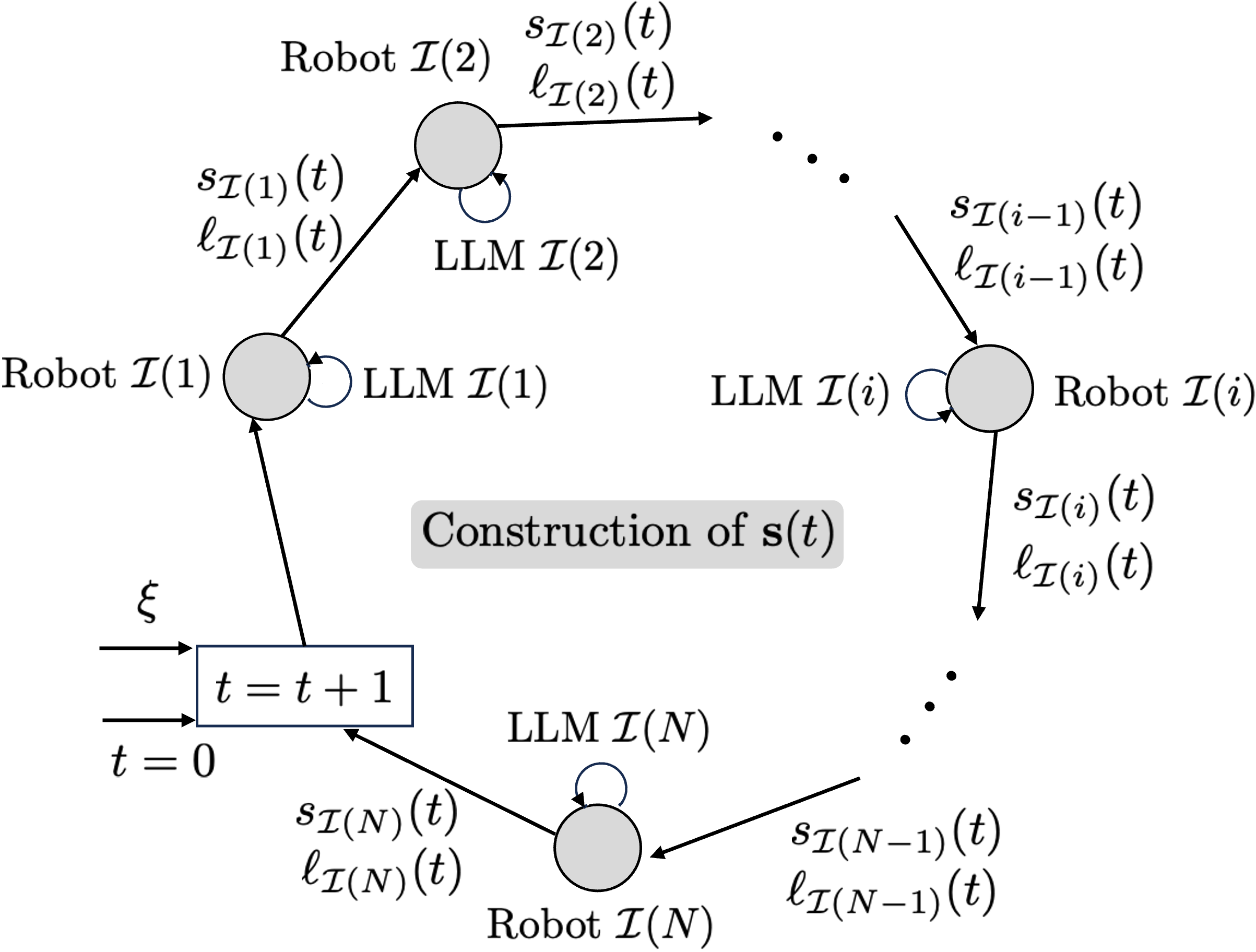
Given a task scenario \(\xi\), the robots select decisions at each time \(t\) sequentially as per an ordered set \(\mathcal{I}\) of robot indices. Each robot \(j = \mathcal{I}(i)\) (gray disk) is delegated to an LLM that generates a decision \(s_j(t)\) given textual context \(\ell_{\mathcal{I}(i-1)}(t)\) containing the task description and past robot decisions provided by the previous robot \(\mathcal{I}(i-1)\). If the LLM for robot \(j\) is not certain enough about what the correct decision \(s_j(t)\) is, the robots seek assistance (not shown).
Citation
Acknowledgements
The website template is from Code as Policies.